Our Services
Search Engine Optimization
We have the expertise to give your business the SEO boost you’re looking for. Learn more about the services we offer below.
What is SEO?
Search engine optimization (SEO) is the process of increasing a website’s search engine rankings to get more high quality traffic from the search engines results. SEO focuses on organic (free) traffic, rather than paid traffic, which generally charge advertisers per impression or click. Search engines vary widely in functionality, specialty, and the results they provide. Many modern search engines offer rich search results that include web pages, images, videos, shopping, academics, news, and maps.
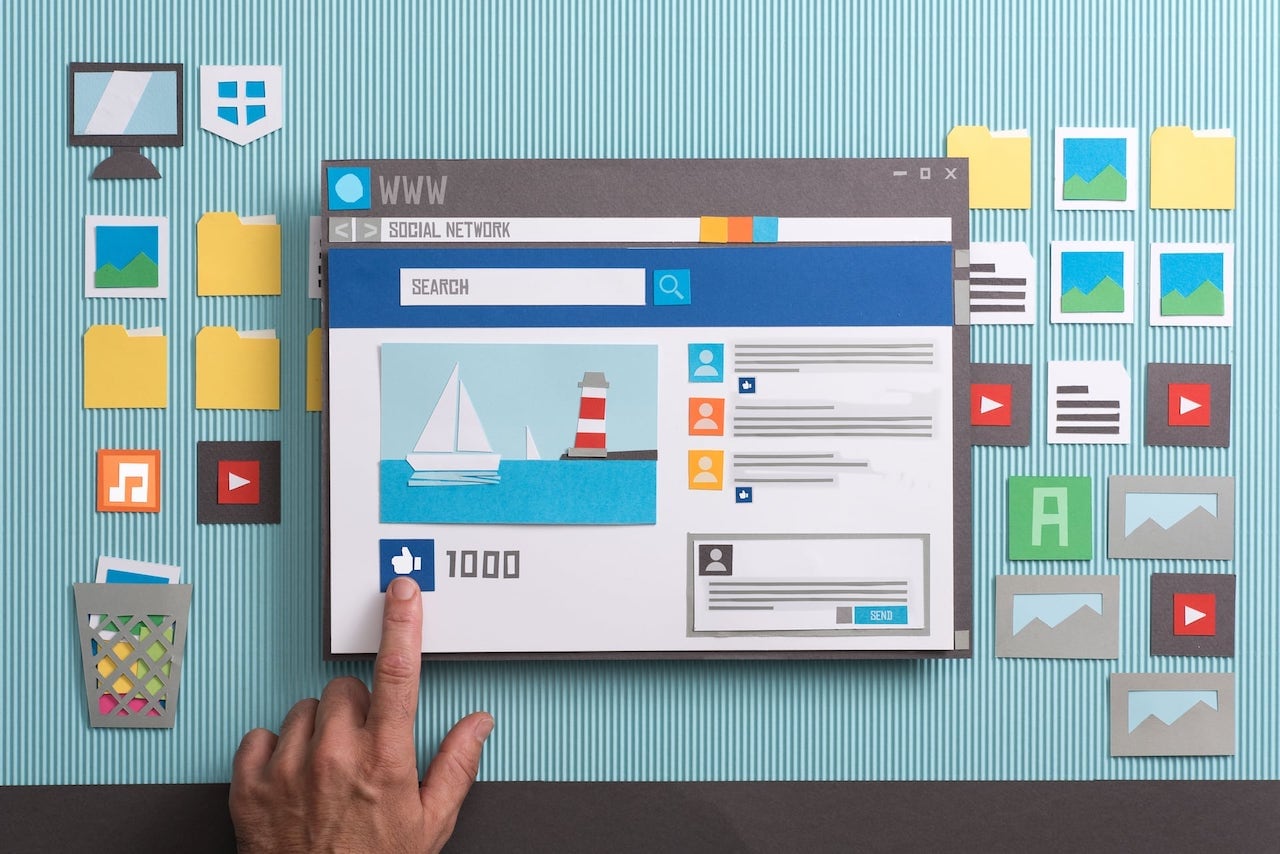
Search Engine Functions
There are three basic functions of a search engine.
- Crawling: Using specialized programs known as crawlers, search engines find and download text, images, videos, etc. from websites it finds on the web.
- Indexing: Search engines scan a page’s contents before storing them in its database, also known as an index.
- Delivering Search Results: When a user conducts a search on a search engine, the search engine returns results that best match their inquiry.
Have Questions?
Learn More About Our Services Now
Find Out if We're a Good Fit for Your Project. Talk to an Expert.
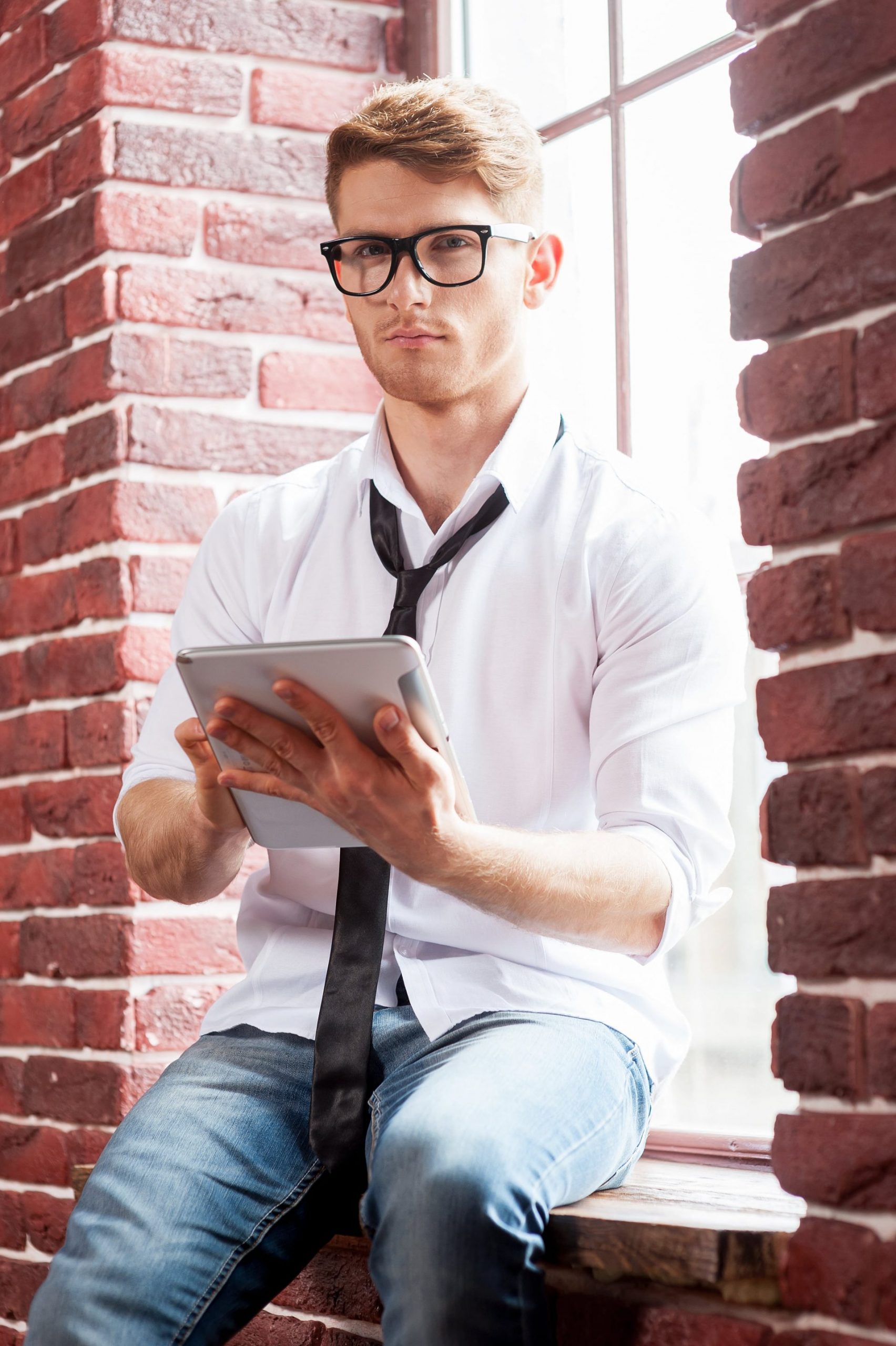
Search Paradigms
There have been several paradigms in search over the past 30 years. The first paradigm, or the first 10 years of search, centered on a query’s relevance to a web page. Although this functioned, it was easily spammed and made prioritization in the search results difficult. Back then searchers would often go back 5-10 pages in the search results to find a suitable website.
The second paradigm, or the second 10 years of search, added a new dimension (“backlinks”) as a way to establish priority in the search results. Led by Google and its famous algorithm, Page Rank, search engines began treating backlinks like votes for web pages which made certain pages more important than others.
The third paradigm, or the third 10 years of search, centered not on relevance or importance, but rather on delivering the best search results to users. Using new technologies, search engines have been able to offer a variety of search result types, and personalize them based on location, history, etc. At present, search engines have even began to include artificial intelligence as a way to better match search results with user intent.
Search Results
Today we’re in the third paradigm of search. We don’t just try to be the most relevant or most important, we try to offer the best search results possible for each keyword we target. That means serving highly optimized pages with:
- Easy Accessibility: Crawlable and indexable
- Relevant Content: Topic, intent, and timeframe
- Valuable Information: Engaging, satisfying, and complete
- Fast Load Times: Core Web Vitals (LCP, FID, and CLS)
- Responsive Design: Desktop, tablet, and mobile
- Secure Connections: SSL/TLS and security headers
At Netmark, we have over 15 years of experience providing search engine optimization services for clients that span the globe. Our team uses a unique combination of science and data to create strategies that help maximize our impact.